Investing in Deep Tech: Opportunity in AI's Bottlenecks
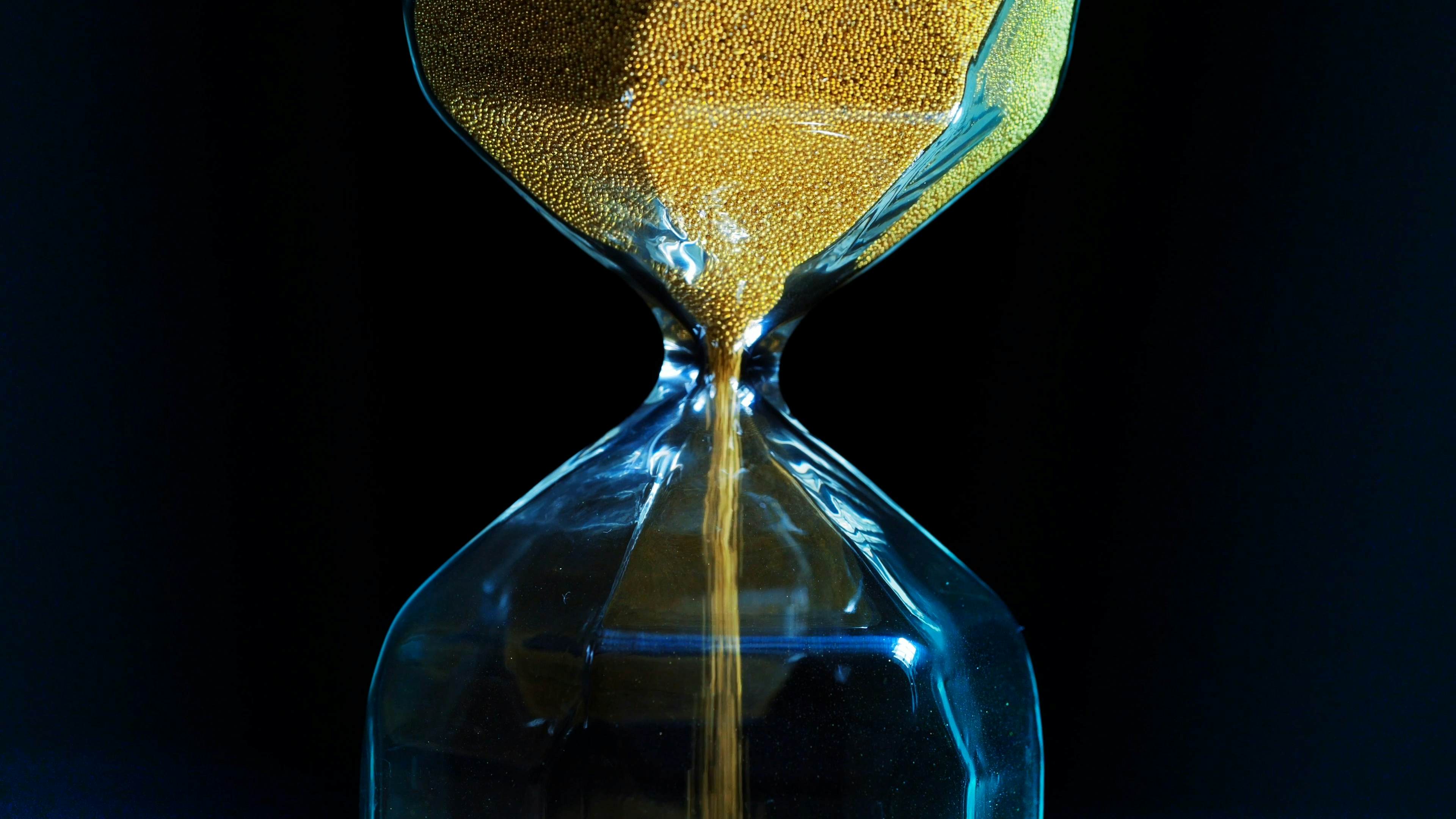
AI ventures startups will have a dual job going forward: innovating/disrupting and compensating for problems that increase as the tech rolls out. That’s not uncommon with deep tech, which focuses on the hardest, most revolutionary technology. These are “big-swing” developments that can take lots of time and money to develop but pay off with major advances for society and handsome returns for venture investors. They are also typically areas where there’s no standing still.
In this article, we explore key bottlenecks in the rapid development of artificial intelligence (AI) and highlight startups already making strides to overcome limitations.
For more on this topic, please join our webinar.
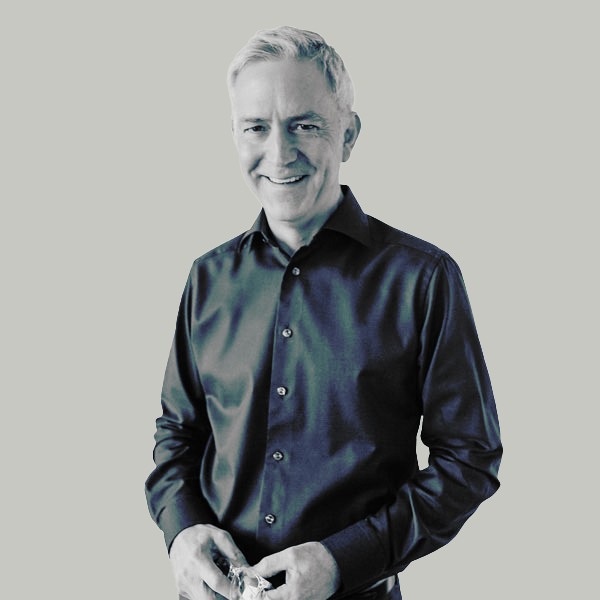
Todd McIntyre
Managing Partner, Deep Tech FundTodd has more than 20 years of experience as a venture capital investor, entrepreneur, and senior technology operating executive, as well as deep knowledge of intellectual property and IP licensing. He has worked with capital structures ranging from seed stage investments to public offerings and has experience in many sectors, including consumer media and web, optoelectronics, telecoms, cleantech, and healthcare. Most recently, he was founding Managing Partner of Grey Sky Venture Partners, a life sciences and digital health fund that pioneered a venture finance model combining early-stage capital with fund-owned intellectual property. He also served as a senior business leader in a technology incubator fund, where he led efforts to build, fund, and spin out several new deep tech businesses. Todd received his BA from Hendrix College and holds an MBA from Stanford Graduate School of Business.
AN EXPLOSIVE TRAJECTORY: THE STATE OF AI
AI is currently experiencing explosive growth, fueled by advancements across computing hardware, algorithm design, and data processing. The market has ballooned from around $100 billion in 2022 to projections nearing $2 trillion by 2030, representing a 20-fold increase in size.
Three primary trends have converged to enable this rapid rise.
1. Machine learning techniques like deep neural networks have become exponentially more sophisticated. Where early AI systems relied heavily on rules-based logic, modern neural networks can infer patterns and make judgments from vast datasets. This greatly expands the tasks AI can perform.
2. Specialized computing hardware has unlocked vastly increased processing to handle AI’s huge computational demands. Modern graphics processing units (GPUs) offer parallelized architectures tailored for neural network calculations, while new system-on-a-chip designs integrate processing, memory, and interconnects for acceleration.
3. The proliferation of smart devices, digital services, and IoT sensors has generated a deluge of data to supply AI systems. The raw material to train algorithms on real-world examples has massively expanded.
Combined, these innovations have progressed AI from narrow applications in the 1990s and 2000s to today’s versatile technology infiltrating nearly every industry. AI now promises to transform major sectors like healthcare, transportation, finance, manufacturing, agriculture, and more by enabling new products, services, and efficiencies.
VISIONARY POTENTIAL: INDUSTRY IMPACT
AI’s versatility arises from its ability to perform an ever-wider range of cognitive functions. Core capabilities like analyzing data for patterns, making predictions, generating new content, communicating in natural language, controlling mechanical systems, and recognizing images allow AI to enhance almost any information-based task.
Healthcare stands out as an industry ripe for AI-driven modernization. Algorithms can rapidly parse patient histories, scans, lab tests, clinical research, and population data to improve diagnostic accuracy, treatment planning, and medical discoveries. AI-guided robotics and proximity sensors also promise to assist overburdened care staff. The net result could be more affordable, preventative, and personalized care.
Transportation and logistics also stand to gain tremendously from AI. Autonomous vehicles could coordinate to optimize traffic flows, reducing congestion and pollution while improving road safety. Smart warehouses with inventory tracking and predictive supply chain analytics may minimize shipping delays and wasted inventory. Overall, AI offers more intelligent, efficient infrastructure.
Even specialized fields like materials science and engineering can progress faster with AI accelerating experiments and discoveries. Algorithms can identify promising new combinations to test based on scientific literature, patents, and chemical databases. Machine learning further helps decipher insights from test results. Less time spent on manual research allows more rapid iteration and innovation.
Across sectors, AI is transforming products, services, and business models. Thus an enormous opportunity exists for investors to fund these potentially transformative technologies. However, fully capitalizing requires analyzing and addressing AI’s scaling bottlenecks.
UNDERSTANDING LIMITS: AI’S KEY BOTTLENECKS
AI faces the challenges of any exponentially new, growing, infrastructurally intensive technology. Understanding these barriers helps identify the most strategic investment opportunities. These are some of AI’s limiters.
1. Computational power. State-of-the-art AI models require enormous computational resources for training and inference. Large language models like GPT-3 boast over 175 billion parameters, demanding specialized hardware accelerators attaching hundreds of graphics cards parallelized across multiple servers. The limitations of current computing platforms curb further growth.
2. Data quality and availability. Another bottleneck is the immense volumes of quality data required to train advanced neural networks. Algorithms learn from exposure to extensive, well-labeled, representative example datasets. However, few processes exist to efficiently collect, clean, label, and share high-quality data at scale.
3. Algorithmic accuracy. Despite rapid gains, AI still falls well short of generalized human cognition. Algorithms remain brittle. Minor input changes can wildly alter outputs and conclusions. Ensuring consistent, accurate predictions across diverse real-world scenarios remains deeply challenging.
4. Real-world applicability. Even where algorithms succeed on isolated tasks, it often proves difficult to implement capabilities into complex operational environments. Customizing models and integrating predictions into business processes involves extensive additional engineering. This means realized value can lag far behind raw technical capability.
OPPORTUNITY IN OBSTACLES: PROMISING VENTURE SPACES
Understanding AI’s key bottlenecks and ecosystem helps spotlight attractive investment spaces. In particular, startups focused on computational hardware, data infrastructure, and practical implementation offer strong prospects.
Specialized Computational Hardware
Maximizing returns on AI requires specialized computing hardware pushing beyond the limits of architectures. Traditional x86 central processing units (CPUs) that power most computers aren’t efficient enough for the parallel workloads of neural networks.
While graphics processing units (GPUs) currently lead for acceleration, their fixed designs have limited configurability. What’s needed is hardware tailored specifically for variable AI models. Promising startup architectural approaches include multi-chip modules, in-memory computing, optical interconnects, and analog designs for efficiency.
Notably, the shortage of advanced computing hardware now heavily constrains access to state-of-the-art AI. Cloud providers like Amazon Web Services report lengthy waiting lists for specialized instances with these accelerators.
In total, the market for AI accelerators is projected to grow over 6X to $50 billion+ by 2025. Startups developing novel hardware have huge potential to prospects to further AI advancement and as investing opportunities.
Data Management Infrastructure
Scalable, high-quality datasets are the critical raw material for developing accurate AI models. However, there are massive costs around data engineering, labeling, and tooling. Startups focused on data management infrastructure can lower these barriers.
Promising areas include:
- Data integration platforms consolidating siloed archives into unified lakes
- Data labeling interfaces and training to scale annotation
- Synthetic data generation to augment real-world examples
- Data debugging tools to assess model readiness
- Data marketplaces and sharing protocols enabling open-sourcing
Notably, emerging data trusts allow collective data sharing while respecting privacy rights. Overall, better data tooling promises to accelerate development and adoption by reducing time and skill bottlenecks. The market for data management could expand from ~$90 billion today to over $230 billion by 2026.
Deployment and Implementation
Finally, bridging the gap between raw AI insights and live systems promises significant challenges and rewards. Taking algorithms from controlled environments into dynamic business contexts requires extensive specialization and customization.
Key enablers spurring adoption include integration tools, monitoring suites, annotation interfaces, customizable modules, and pre-trained models. In addition, consulting firms can help develop and implement solutions tailored to individual use cases and technical environments.
In total the AI implementation market could grow from around $10 billion presently to $60 billion by 2030. Startups facilitating this last-mile adoption across industries have strong prospects as demand rapidly increases.
NOTABLE STARTUPS TARGETING SOLUTIONS
Many promising startups directly address AI’s bottlenecks across hardware, data, and implementation. Here are a few venture-backed startups developing transformative solutions.
Groq: Specialized AI Processing Units
Groq has developed an innovative processing architecture optimized for accelerating machine learning models. Their Tensor Streaming Processor design leverages a single instruction set architecture that can be programmed and updated dynamically based on workload. This differs profoundly from multi-core, fixed-function accelerators like GPUs.
Flexibility allows software-based optimization and customization to maximize performance across different models and datasets. Efficiency at scale reaches 1,000 TOPS per processor — a huge increase vs. alternatives. Groq’s focus on ease of deployment via PCIe cards in standard servers is another benefit..
By specializing hardware for variable AI workloads beyond the limits of repurposed GPUs, Groq promises to push machine learning capabilities forward. Its recent $367M Series C funding highlights strong market interest.
Legion: Data-Labeling Platform
Successfully training AI relies on extensive labeled demonstration data. However, manually annotating images, videos, text, or audio at scale is very costly. Startup Legion provides data labeling tooling to lower these barriers through economies of scale and annotation process enhancements.
Centralization onto Legion’s platform enables pooling labeling task allocation across clients to smooth workflows and better utilize expert labelers. Streamlined interfaces reduce time on tasks through smart workflows and productivity trackers. Automated validation, quotas, and testing reduce errors. Integration of modeling helps suggest additional useful labels. Overall, Legion accelerates and enhances annotation, which is critical for quality training data.
Having banked over $100M in funding, Legion’s success spotlights the need for solutions to data bottlenecks.
Mainspring: Specialized AI Infrastructure
Data centers hosting AI applications demand reliable, sustainable power to support intense computation. Mainspring’s advanced linear generator technology provides an innovative solution. Their fuel-flexible system allows low-emission operation on combinations of natural gas, hydrogen, and biogas. Importantly, this independence from the grid increases reliability for continuous AI workloads.
Recent major partnerships, like a large generator supply contract from leading renewable energy producer NextEra, demonstrate market appetite. Mainspring’s generators could become integral infrastructure enabling uninterrupted, efficient AI advancement.
JOIN OUR WEBINAR
AI currently faces critical technology and infrastructure barriers. Strategic investment in supplementary hardware, data, and operationalization systems promises to push boundaries further. Getting behind the right ventures providing these solutions has the potential for generational rewards.
If you’d like to learn more about the obstacles and opportunities, please join our upcoming webinar on the topic. Sign up here.